Context Data vs. Dynamiq
Context Data
contextdata.ai/Context Data is an enterprise data infrastructure built to accelerate the development of data pipelines for Generative AI applications. The platform automates the process of setting up internal data processing and transformation flows using an easy-to-use connectivity framework where developers and enterprises can quickly connect to all of their internal data sources, embedding models and vector database targets without having to set up expensive infrastructure or engineers.
Dynamiq
www.getdynamiq.ai/Dynamiq the operating platform for building, deploying, monitoring and fine-tuning generative AI applications. Key features: 🛠️ Workflows: Build GenAI workflows in a low-code interface to automate tasks at scale 🧠 Knowledge & RAG: Create custom RAG knowledge bases and deploy vector DBs in minutes 🤖 Agents Ops: Create custom LLM agents to solve complex task and connect them to your internal APIs 📈 Observability: Log all interactions, use large-scale LLM quality evaluations 🦺 Guardrails: Precise and reliable LLM outputs with pre-built validators, detection of sensitive content, and data leak prevention 📻 Fine-tuning: Fine-tune proprietary LLM models to make them your own
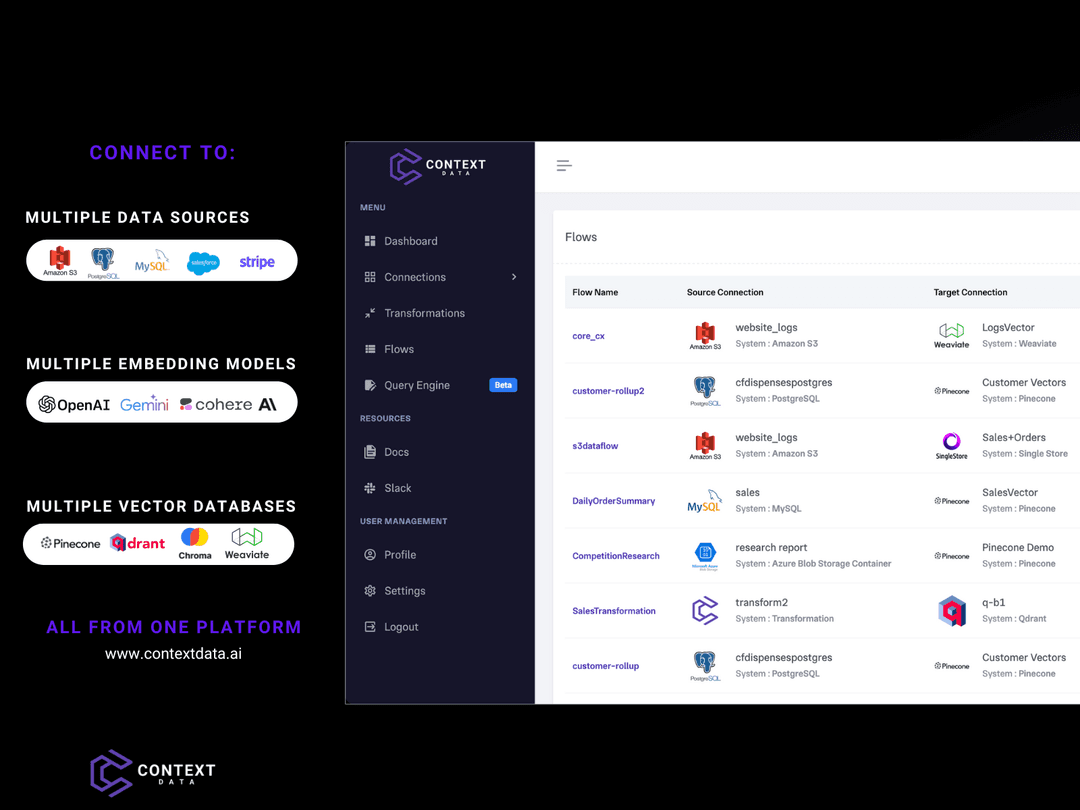
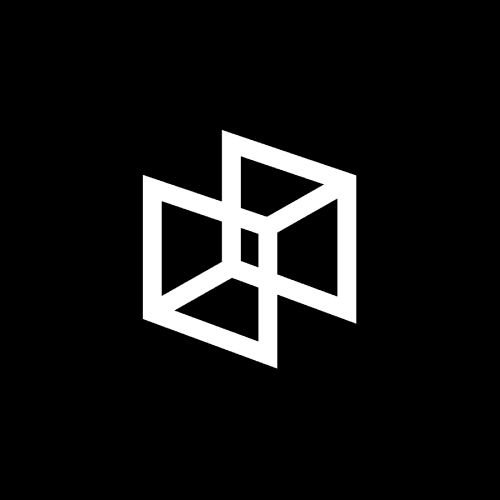
Top Reviews
@far-walrus-09
For startups and enterprise companies that are building internal Generative AI solutions, Context Data automates the process and time to deploy data platforms from an average of 2 weeks to less than 10 minutes and at 1/10th of the cost.
@far-walrus-09
Context Data is a Data Processing & ETL infrastructure for Generative AI applications.
Rankings
Pros
- Multi-Source Transformations× 1
- One-Click Model Connections× 1
- Smart Scheduling× 1
Cons
Pros
Cons
Frequently Asked Questions
Context Data is ideal for enterprises and startups looking to accelerate the development of data pipelines for Generative AI applications. It automates the setup of internal data processing and transformation flows, significantly reducing deployment time and cost. Key advantages include multi-source transformations, one-click model connections, and smart scheduling. On the other hand, Dynamiq offers a comprehensive platform for building, deploying, monitoring, and fine-tuning Generative AI applications. It features low-code workflows, custom LLM agents, and advanced security measures. Dynamiq is vendor-agnostic, allowing flexibility in model choices. The better platform depends on whether you prioritize rapid data pipeline setup (Context Data) or an all-in-one, secure solution with extensive customization options (Dynamiq).
Dynamiq offers more customization options compared to Context Data. Dynamiq allows users to build custom GenAI workflows, create custom LLM agents, and fine-tune proprietary LLM models. It also supports the creation of custom RAG knowledge bases and provides extensive observability and guardrails for reliable outputs. Context Data, while efficient in setting up data pipelines quickly with features like multi-source transformations and one-click model connections, does not offer the same level of customization as Dynamiq.
Context Data is designed to be highly cost-effective, reducing the time to deploy data platforms from an average of 2 weeks to less than 10 minutes at a tenth of the cost. This makes it a particularly attractive option for startups and enterprises focused on minimizing expenses in the initial setup of data processing and transformation flows. Dynamiq, while offering a wide range of features and extensive customization, does not specifically highlight cost reduction as a primary benefit. Therefore, Context Data is likely the more cost-effective option.
Dynamiq is better suited for enterprises managing highly sensitive data due to its stringent security controls and air-gapped solution. These features enable clients to leverage LLMs while maintaining high levels of data security. Context Data, while efficient and cost-effective, does not emphasize security measures to the same extent as Dynamiq. Therefore, if data security is a critical concern, Dynamiq would be the better choice.
Both platforms aim to streamline and accelerate the deployment of Generative AI solutions, but they do so in different ways. Context Data reduces the time to deploy data platforms from an average of 2 weeks to less than 10 minutes, focusing on automating data processing and transformation flows. Dynamiq, on the other hand, offers low-code interfaces for building workflows and deploying custom LLM agents, which can also speed up deployment times. However, Context Data is specifically optimized for rapid data pipeline setup, making it particularly effective for quickly deploying Generative AI solutions.
Context Data is an enterprise data infrastructure designed to accelerate the development of data pipelines for Generative AI applications. It automates the setup of internal data processing and transformation flows using an easy-to-use connectivity framework. This allows developers and enterprises to quickly connect to all of their internal data sources, embedding models and vector database targets without the need for expensive infrastructure or engineers.
Pros of Context Data include Multi-Source Transformations, One-Click Model Connections, and Smart Scheduling. Currently, there are no user-generated cons listed for Context Data.
Context Data automates the process and time to deploy data platforms for startups and enterprise companies building internal Generative AI solutions. It reduces the deployment time from an average of 2 weeks to less than 10 minutes and cuts the cost to 1/10th of the traditional expense.
Context Data provides a Data Processing & ETL infrastructure specifically designed for Generative AI applications.
Dynamiq is an operating platform designed for building, deploying, monitoring, and fine-tuning generative AI applications. It offers a variety of features including low-code workflow automation, custom knowledge base creation, LLM agent operations, observability, guardrails for reliable outputs, and fine-tuning of proprietary LLM models.
The key features of Dynamiq include: - Workflows: Build GenAI workflows in a low-code interface to automate tasks at scale. - Knowledge & RAG: Create custom RAG knowledge bases and deploy vector DBs in minutes. - Agents Ops: Create custom LLM agents to solve complex tasks and connect them to your internal APIs. - Observability: Log all interactions and use large-scale LLM quality evaluations. - Guardrails: Ensure precise and reliable LLM outputs with pre-built validators, detection of sensitive content, and data leak prevention. - Fine-tuning: Fine-tune proprietary LLM models to make them your own.
The benefits of using Dynamiq include: - Air-gapped Solution: Enables clients managing highly sensitive data to leverage LLMs while maintaining stringent security controls. - Vendor-Agnostic: Allows clients to build GenAI applications using a variety of models from different providers and switch between them if needed. - All-In-One Solution: Covers the entire GenAI development process from ideation to deployment.
The use cases for Dynamiq include: - AI Assistants: Equip teams with custom AI assistants to streamline tasks, enhance information access, and boost productivity. - Knowledge Base: Build a dynamic AI knowledge base to streamline decision-making and enhance productivity by reducing the time spent navigating through extensive company documents, files, and databases. - Workflow Automations: Design powerful, no-code workflows to enhance content creation, CRM enrichment, and customer support.
As of now, there are no user-generated pros and cons for Dynamiq. However, its key benefits include stringent security measures, vendor-agnostic integration capabilities, and an all-in-one solution for GenAI development.